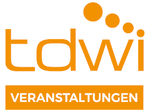
Please note:
Here you can find the English speaking sessions of the TDWI München 2024. You can find all conference sessions, including the German speaking ones, here.
Data Architecture to Support Analytics & Data Science
Supporting analytics and data science in an enterprise involves more than installing open source or using cloud services. Too often the focus is on technology when it should be on data. The goal is to build multi-purpose infrastructure that can support both past uses and new requirements. This session discusses architecture principles, design assumptions, and the data architecture and data governance needed to build good infrastructure.
Target Audience: BI and analytics leaders and managers; data architects; architects, designers, and implementers; anyone with data management responsibilities who is challenged by recent changes in the data landscape
Prerequisites: A basic understanding of how data is used in organizations, applications and platforms in the data ecosystem, data management concepts
Level: Advanced
Extended Abstract:
The focus in our market has been on acquiring technology, and that ignores the more important part: the landscape within which this technology exists and the data architecture that lies at its core. If one expects longevity from a platform then it should be a designed rather than accidental architecture.
Architecture is more than just software. It starts with uses, and includes the data, technology, methods of building and maintaining, governance, and organization of people. What are design principles that lead to good design and data architecture? What assumptions limit older approaches? How can one modernize an existing data environment? How will this affect data management? This session will help you answer these questions.
Outline - Topics covered:
- A brief history of data infrastructure and past design assumptions
- Categories of data and data use in organizations
- Differences between BI, analysis, and data science workloads
- Data architecture
- Data management and governance processes
- Tradeoffs and antipatterns
Mark Madsen works on the use of data and analytics for decision-making and organizational systems. Mark worked for the past 25 years in the field of analytics and decision support, starting with AI at the University of Pittsburgh and robotics at Carnegie Mellon University.