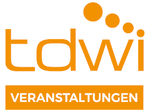
Please note:
On this site, there is only displayed the English speaking sessions of the TDWI München digital. You can find all conference sessions, including the German speaking ones, here.
The times given in the conference program of TDWI München digital correspond to Central European Time (CET).
By clicking on "EVENT MERKEN" within the lecture descriptions you can arrange your own schedule. You can view your schedule at any time using the icon in the upper right corner.
ROOM K4 | Operationalizing Machine Learning in the Enterprise
What does it take to operationalize machine learning and AI in an enterprise setting? This seems easy but it is difficult. Vendors say that you only need smart people, some tools, and some data. The reality is that to go from the environment needed to build ML applications to a stable production environment in an enterprise is a long journey. This session describes the nature of ML and AI applications, explains important operations concepts, and offers advice for anyone trying to build and deploy such systems.
Target Audience: analytics manager, data scientist, data engineer, architect, IT operations
Prerequisites: Basic knowledge of data and analytics work
Level: Basic
Extended Abstract:
What does it take to operationalize machine learning and AI in an enterprise setting?
Machine learning in an enterprise setting is difficult, but it seems easy. You are told that all you need is some smart people, some tools, and some data. To travel from the environment needed to build ML applications to an environment for running them 24 hours a day in an enterprise is a long journey.
Most of what we know about production ML and AI come from the world of web and digital startups and consumer services, where ML is a core part of the services they provide. These companies have fewer constraints than most enterprises do.
This session describes the nature of ML and AI applications and the overall environment they operate in, explains some important concepts about production operations, and offers some observations and advice for anyone trying to build and deploy such systems.
Mark Madsen is a Fellow in the Technology & Innovation Office at Teradata where he works on the use of data and analytics to augment human decision-making and evolve organizational systems. Mark worked for the past 25 years in the field of analytics and decision support, starting with AI at the University of Pittsburgh and robotics at Carnegie Mellon University. He is also on the faculty of TDWI.
Vortrag Teilen